Ethical dilemmas arise from predictive student analytics
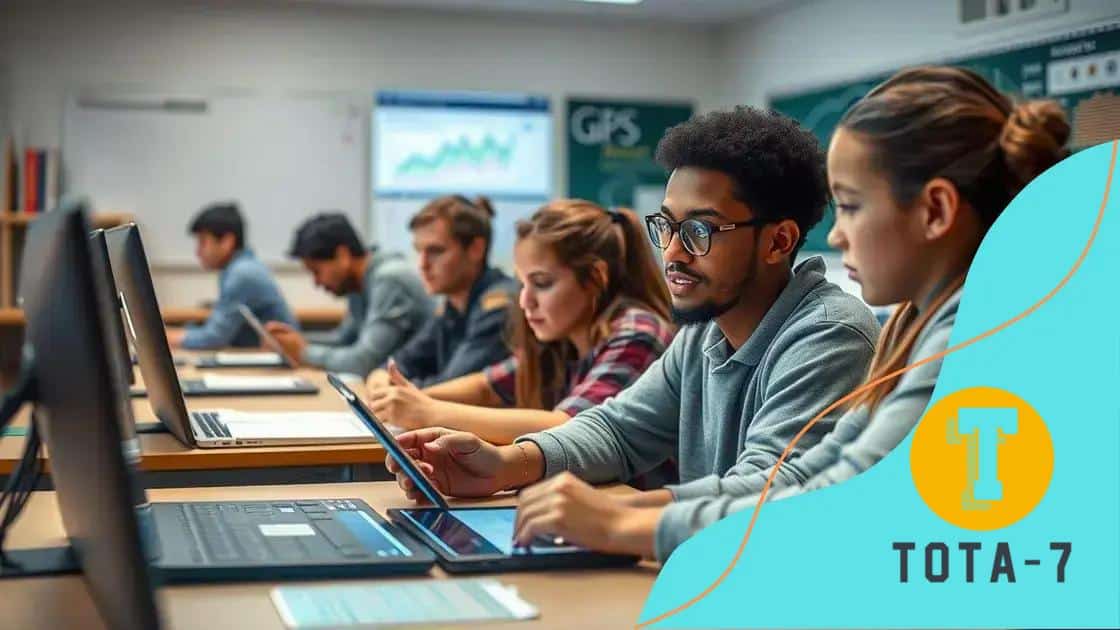
Ethical dilemmas arise from predictive student analytics as they can improve educational outcomes but also lead to biases and inequities if not implemented responsibly.
Ethical dilemmas arise from predictive student analytics, challenging educators and institutions. Have you ever thought about how data influences student success and concerns regarding its use? Let’s delve in!
Understanding predictive analytics in education
Understanding predictive analytics in education is essential for enhancing student outcomes and improving institutional effectiveness. This technology uses data to anticipate future performance and outcomes. Educators can harness these insights to tailor their approaches and foster better learning environments.
What is Predictive Analytics?
Predictive analytics involves statistical techniques and machine learning algorithms to analyze historical data and make forecasts. In the education sector, it can predict which students might struggle, helping teachers intervene early. By analyzing various factors, from attendance to grades, institutions can effectively utilize resources.
Key Benefits
- Improved student support: Early identification of students at risk can lead to timely intervention.
- Tailored learning experiences: Analytics allow for customization in teaching methods to suit individual needs.
- Enhanced decision-making: Data-driven insights help educators and administrators make informed choices.
Moreover, understanding how to implement predictive analytics responsibly is crucial. Data privacy and ethical considerations must guide decision-making. Institutions need to ensure that they are using student data appropriately and transparently to maintain trust.
A collaborative approach among educators, data specialists, and policy-makers is vital for successful implementation. Educators should be trained to work with data effectively, while also keeping the ethical implications at the forefront. When done right, predictive analytics can transform the educational landscape, paving the way for academic success.
Privacy concerns in student data usage
Privacy concerns in student data usage are a significant issue in today’s educational landscape. With the rise of technology, schools are collecting vast amounts of student data, which can lead to questions about how it is stored and used. It’s vital to understand these concerns deeply.
Importance of Data Privacy
Protecting student data is essential for maintaining trust between students, parents, and educational institutions. When data is misused or exposed, it can lead to serious consequences. Schools must prioritize data security measures to protect sensitive information.
Common Privacy Issues
- Informed consent: Students and parents often need to understand what data is collected and how it will be used.
- Data breaches: Schools must be prepared to prevent unauthorized access to student information.
- Use of third-party services: Many educational tools require sharing data, raising questions about where this information is stored and how it is protected.
Additionally, schools must ensure compliance with laws such as FERPA, which regulates the access and sharing of student data. Educators should be mindful of how data analytics can impact student privacy. Discussions around ethical use of data are crucial.
Training educators on best practices regarding data privacy can help mitigate risks. Encouraging open conversations about data usage can also create a culture of transparency, allowing everyone to feel safer about their information.
The impact on student equality and fairness
The impact of predictive analytics on student equality and fairness is a critical discussion in education today. This technology can help identify students who may need additional support, but it can also deepen existing inequalities if not applied carefully.
Understanding Equity in Education
Equity means ensuring that all students have access to the resources and opportunities they need to succeed. When predictive analytics is misused, it can lead to biased outcomes based on race, socioeconomic status, or other factors. This is why careful consideration is essential in its deployment.
Potential Challenges
- Data bias: If the data used for analysis reflects existing biases, the predictions may favor certain groups over others.
- Access to resources: Students from underprivileged backgrounds may not receive the same level of support, leading to wider achievement gaps.
- Transparency issues: Educators and administrators must be clear about how data is collected and used to avoid misunderstandings.
Moreover, the algorithms used to analyze data play a vital role in determining fairness. If schools do not regularly evaluate these algorithms for bias, they risk perpetuating inequality. Open discussions about data privacy and ethical usage must be part of the mission in educational institutions.
Equity cannot simply be a goal; it must be a practice. Schools need to actively engage in providing equitable resources and opportunities for all students. This could include targeted interventions where data indicates a need.
Addressing biases in predictive models
Addressing biases in predictive models is crucial for ensuring fairness in education. These models can significantly affect student outcomes, which is why it’s essential to analyze and correct any biases present in the data.
The Nature of Bias in Predictive Models
Bias in predictive models often stems from the data used to train them. If the training data reflects societal inequalities, the model will likely reproduce those biases. For instance, if historical data shows that certain groups of students have lower success rates, the model might unfairly predict that current students from those groups will also struggle.
Steps to Address Bias
- Data Audits: Regularly auditing the data used for predictive models can help identify biases. This includes checking for representation of diverse student groups.
- Inclusive Data Collection: Efforts should be made to collect data that accurately reflects the diverse backgrounds of all students. This can improve model predictions.
- Algorithm Transparency: Schools should strive to use algorithms that allow for understanding and explanation of how decisions are made, making it easier to identify potential biases.
Additionally, collaboration among educators, data scientists, and policy-makers can improve the effectiveness of predictive models. Continuous feedback loops can help refine the models to be more equitable. Educational institutions should also involve students and parents in discussions about how data is utilized.
Implementing these strategies can help mitigate bias and promote fairness in educational outcomes. By ensuring that predictive models are transparent and equitable, schools can better support all students.
Strategies for ethical implementation of analytics
Strategies for the ethical implementation of analytics in education must focus on transparency and fairness. As predictive analytics becomes more prevalent, educational institutions have a responsibility to use these tools ethically.
Developing Ethical Guidelines
Creating a clear set of ethical guidelines for data use is essential. These guidelines should define how data is collected, who has access, and how it will be used. Making this information accessible to students and parents helps build trust.
Best Practices for Data Use
- Transparency: Schools should communicate openly about the data they collect and how it influences students’ learning experiences.
- Informed consent: Educators must ensure that students and parents are aware of data collection practices and agree to them.
- Continuous training: Providing training for educators on ethical data practices can promote responsible use of analytics in the classroom.
Additionally, it’s important to implement regular audits of analytics practices. By reviewing how data is used, schools can identify any potential ethical issues and adjust their practices. Engaging stakeholders from various backgrounds in these reviews will ensure diverse perspectives are considered.
Furthermore, promoting a culture of accountability within schools helps in maintaining ethical standards. When teachers and administrators understand the importance of responsible data use, they are more likely to adhere to ethical practices.
In conclusion, using predictive analytics in education can be a powerful tool for enhancing student learning and success. However, it’s vital to address ethical concerns to ensure that all students are treated fairly. By focusing on transparency, informed consent, and continuous training, schools can create a data-driven culture that benefits everyone. Listening to stakeholders and regularly assessing analytics practices will help institutions to implement strategies that prioritize equity and accountability. As we move forward, ethical considerations must remain at the forefront of discussions around student data and analytics.
FAQ – Frequently Asked Questions about Predictive Analytics in Education
What are predictive analytics in education?
Predictive analytics in education involves using data and algorithms to identify patterns and predict student outcomes, helping educators tailor interventions.
How can schools ensure ethical data use?
Schools can ensure ethical data use by creating guidelines for transparency, obtaining informed consent, and regularly auditing data practices.
What is the impact of bias in predictive models?
Bias in predictive models can lead to unfair predictions that affect student opportunities, highlighting the need for diverse data and transparency in algorithms.
Why is stakeholder engagement important in analytics?
Stakeholder engagement is important because it ensures that diverse perspectives shape data practices and policies, promoting equity and accountability.